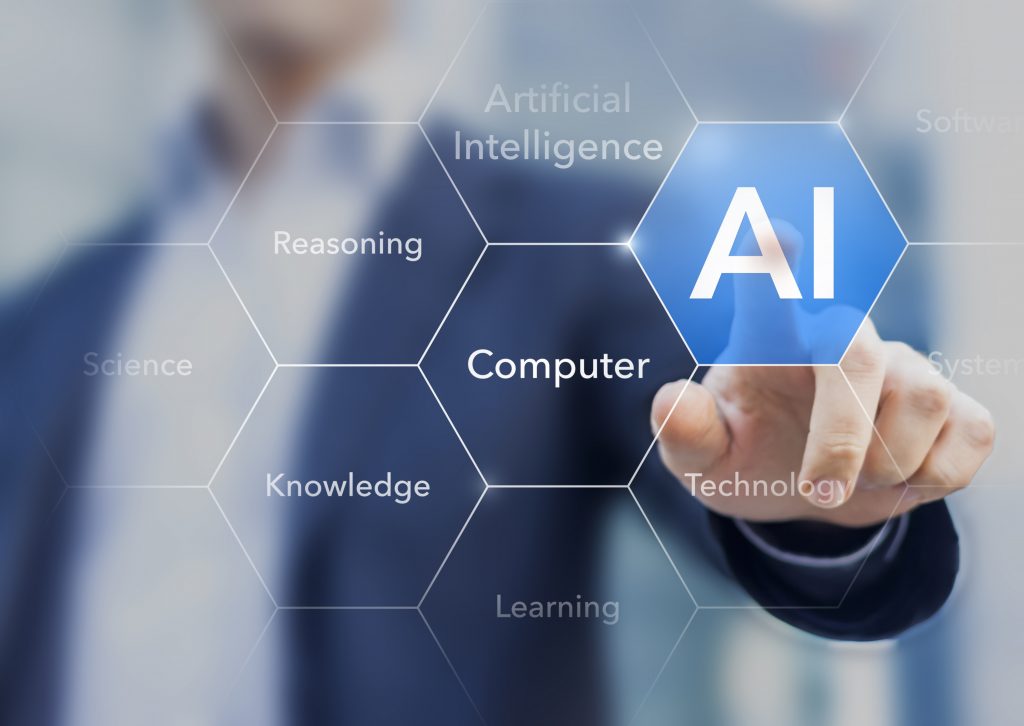
If there has been any time in human history where technological advances in computing have come as fast and furious as they have recently, we’re not aware of it and likely you aren’t either. What comes with so much promise also comes with some level of trepidation for people, but that has always been the case with any type of game-changer advance that’s been seen in human society. A.I. is a great example where we wouldn’t even think about slowing the speed of change it is promising but at the same time most of us hope this doesn’t go sideways in any regard.
But one aspect of this where the potential positive ramifications absolutely must be encouraged is with making better use of data. This is something that any Canadian web hosting provider will be able to relate to, and here at 4GoodHosting we are no different. We understand the magnitude and potential for data management, but can also relate to a lot of shortcomings in how this data is understood and utilized properly.
Which leads us to the topic at hand here today. Advances in AI research have made it so that the use of computer algorithms to differentiate patterns from noise in data is entirely possible, and we now have open-source software and many data scientists streaming into the field. All of this leads to the belief that computer science, statistics, and information technology can lead to a successful AI project with useful outcomes. Teaching humans to think like AI will have value, but will have more of it is teaching AI to understand the value of humans.
Deep – and Deeper – Learning for Neural Networks
One of the most notable ways deep learning neural networks are being put to use is in healthcare, and specifically with more accurately predicting health outcomes. What we are seeing now is state-of-the-art AI algorithms for predicting health outcomes fairly accurately. This could be for whether a patient should be readmitted to the hospital following a surgery, or any number of other very significant decisions that can be better made by AI and its modeling as compared to what a doctor might advise.
While it is true that the deep learning models performed better than some standard clinical models, they still came up short in regards to logistic regression, a widely used statistical method. This suggests that AI learning does have limitations, but it seems the consensus those limitations are a) not significant enough to scale back deployment, and b) likely addressed in future advances with the technology.
Use of AI in healthcare is a great example of how the technology projects, so we’ll stick with it for now. There are indeed limitations, and to address them the early days of this roll out had the readers harmonizing all the data and feeding it into a deep learning algorithm before endeavouring to make sense of it. Some factors that may not be useful for clinical intervention weren’t included, and primarily because they can’t be changed.
The factors that were prioritized for incorporation into neural network architecture were the ones that would improve the performance and the interpretability of the resulting predictive models. Still, until recently there was less of a focus on how all of this would work when dealing with the human operation end of the equation, and that’s where the focus needs to be now.
Advantage to Human Side of AI
Human involvement with an AI project needs to start with a programmer or engineer formulating the question the AI is to address. Let’s say right off the bat that AI will not be able to master this anytime soon. It will require depth, breadth, and synthesis of knowledge of different kinds and AI simply isn’t there yet. Imaging what is missing or what is wrong from what is known is – at least for now – very difficult for modern AIs to do.
Humans are also needed for knowledge engineering. This part of it has been important in the AI field for decades and the current focus is on domain-specific knowledge in the right format to the AI. Reason being so that it doesn’t need to start from scratch when solving a problem. As powerful as AI can be, we should remember that humans have an ability to synthesize knowledge that far exceeds what any computer algorithm can do. That right there is the crux of why valuable human input and reciprocal learning is going to be absolutely essential if AI is going to do what we hope it will.
As AI moves ever forward in being a big part of our lives, it is important to remember that users and those who stand to benefit from it have a key role to play in the data science process attached to the development of it. Done right it will improve the results of an AI implementation and reduce the potential for harm or misuse.